GeoWerkstatt-Project of the Month July 2022
Project: Modeling of ionospheric irregularities using deep learning
Researcher: Alireza Atabati (K. N. Toosi University Teheran; 2022: Guest Researcher at the Institute of Geodesy/Working Group Prof. Dr.-Ing. Jakob Flury)
The ionosphere is the upper part of the Earth's atmosphere, extending from approximately 60 km to 2,000 km, with the main concentration of particles between 300 to 400 km. The interaction of solar radiation with atoms and molecules in the Earth's upper atmosphere causes electron generation in the ionosphere. The ionosphere is a complicated medium. It varies with different parameters, which cause variations in the spatial and temporal structure of electron density and the ionospheric layers. The sun activities’ variations can cause ionospheric electron density irregularities and, thereupon, plasma perturbations, in turn creating fluctuations within this region known as ionospheric irregularities. Ionospheric scintillations are a typical example of such irregularities. This phenomenon usually occurs in the latitude range of 15 degrees to 20 degrees from the magnetic equator, which is called the equatorial ionospheric anomaly, and usually has an electron density greater than that of other regions. In general, ionospheric irregularities can appear at equatorial latitudes besides the mentioned low latitudes because the perturbations in the ionospheric F-layer are more responsible for scintillations initially generated around the magnetic field equator. Ionospheric plasma bubbles are initially generated (generally after sunset) at the F-region bottom side around the magnetic equator, which rise to higher altitudes and extend along magnetic field lines to low latitudes. This increase is related to an unstable process called Rayleigh–Taylor, which leads to disturbances in the ionosphere. The depleted plasma, which contributes to the bubbling process, is combined with the condensed and non-reacted plasma produced in the equatorial ionosphere, creating a strong gradient in the ionospheric structure.
Ionospheric irregularities originate destructive effects on radio signals transmitted from global navigation satellite systems (GNSS). This phenomenon can generate fluctuations in the signal intensity transmitted from the satellite by decreasing the signal-to-noise ratio of the transmitted wave. This is known as amplitude scintillation. Amplitude scintillation can reduce the level of the transmitted signal's lock threshold or cause deep signal fades from the satellite in the case of severe ionospheric scintillation. During ionospheric scintillation, this phenomenon causes a signal deviation in random directions around the main axis of the wave propagation by creating disturbances in the refractive index of the medium. The effect of the ionospheric irregularities on the carrier phase causes cycle slips, disconnects the receiver signal, and leads to the loss of lock. Phase ionospheric scintillation can create a frequency shift in the signal and increase the amount of bandwidth, which locks the receiver's phase and reduces the accuracy of the receiver's ability to connect and lock on to the signal transmitted from the satellite. Accordingly, this process increases the accuracy of the geometric dilution of the precision parameter and thus raises the error rate in determining the position of the receiver, consequently reducing the ability of the receiver to receive and track the transmitted signals.


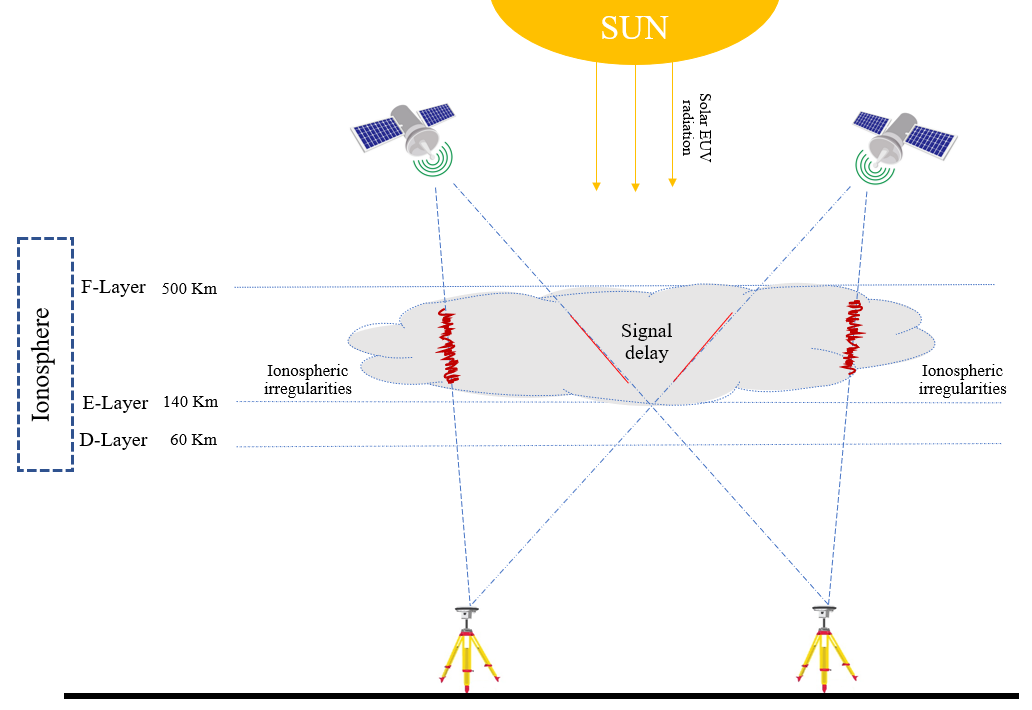
Generally, modeling and predicting ionospheric scintillation can reduce the effects of its error in the observations of GNSS and, therefore, increase the accuracy of satellite communication and navigation application. While multi-frequency observations can almost completely reduce ionospheric delay, they cannot detect or eliminate scintillated signals. Solar activity is primarily responsible for both errors due to disturbances in the ionosphere. Alternatively, plasma instabilities and the electrodynamic process are the primary contributors to ionospheric irregularities. The prediction of ionospheric irregularities using mathematical models is of great importance as these methods can be used to model these phenomena by empirical parameters. Although some empirical models have used parameters such as latitude and longitude, seasons, and time of observation as input, the ionospheric scintillation is affected by physical parameters of the ionosphere, in addition to spatial parameters.
In this research, Hybrid Deep Learning algorithms will be proposed to predict ionospheric irregularities that consist of the combination of Deep Neural Networks (DNN) methods with intelligent optimization techniques such as the Genetic Algorithm (GA) and Particle Swarm Optimization (PSO). The Convolutional Neural Network (CNN) hybrid models stacked over Long Short-Term Memory (LSTM) and Gated recurrent unit (GRU) were proposed to predict local spatiotemporal features from the ionospheric irregularities data sets. The proposed Hybrid DNN models design includes defining the network input data, which contains geophysical data related to ionospheric perturbations, the multi-dimensional convolutional layer, stacked recurrent layers LSTM/GRU structure, and dense layers. The LSTM and GRU structure accept a sequential data form as input, covering a time window of varying duration. In DNN, the initial weights are given randomly. However, using intelligent optimization techniques such as the GA and PSO, these weights are entered into the DNN as initial weights close to the absolute minimum.
Concluding, the Hybrid DNN method integrated with the GA and PSO can detect and predict ionospheric scintillation occurrence near real time on a daily basis, which is critical for reducing the error of the ionospheric irregularities impact on satellite-based communication and navigation system performance. This method can play a precautionary role for alerting GNSS users that the observation epoch might be disturbed by ionospheric irregularities, and GNSS users can eliminate error-infected observations from the dataset.